Machine Learning and Deep Learning: Unleashing the Power of Data
In this data-driven world, businesses are constantly seeking innovative technologies to extract valuable insights and drive growth. Two such technologies, machine learning and deep learning, have gained significant attention for their ability to harness the power of artificial intelligence. In this blog, we investigate the similarities and differences between machine learning and deep learning to explore how both can empower your organization through the power of data.
Machine Learning: Unraveling Patterns and Predictions
Machine learning is an essential branch of artificial intelligence focused on enabling computers to learn and make predictions without being explicitly programmed. It involves the development and application of algorithms that automatically learn from and analyze data to uncover patterns, relationships, and trends. For instance, machine learning powers personalized recommendations on e-commerce platforms and detects fraudulent activities in financial transactions.
Machine learning algorithms work by training on historical data (which is sometimes labeled), extracting relevant features, and then using this knowledge to make accurate predictions on new, unseen data. This “training data” is a stepping stone for the algorithm to uncover underlying patterns, so it can predict real-world data. This data-driven approach has revolutionized automation and decision-making across various industries, including finance, engineering, and manufacturing.
Machine learning can be trained using deep models, also known as deep learning; so, for the purposes of this blog, when we talk about machine learning, we’re specifically referring to non-deep machine learning.
Deep Learning: Unleashing the Power of Neural Networks
Deep learning is a subset of machine learning—simply put, all deep learning is machine learning, but not all machine learning is deep learning. It is focused on building complex artificial neural networks resembling the human brain. These neural networks consist of multiple layers of interconnected nodes, or “neurons,” to process raw data and extract hierarchical representations. Through neural networks, deep learning algorithms can automatically learn intricate patterns and extract rich insights from unstructured data types.
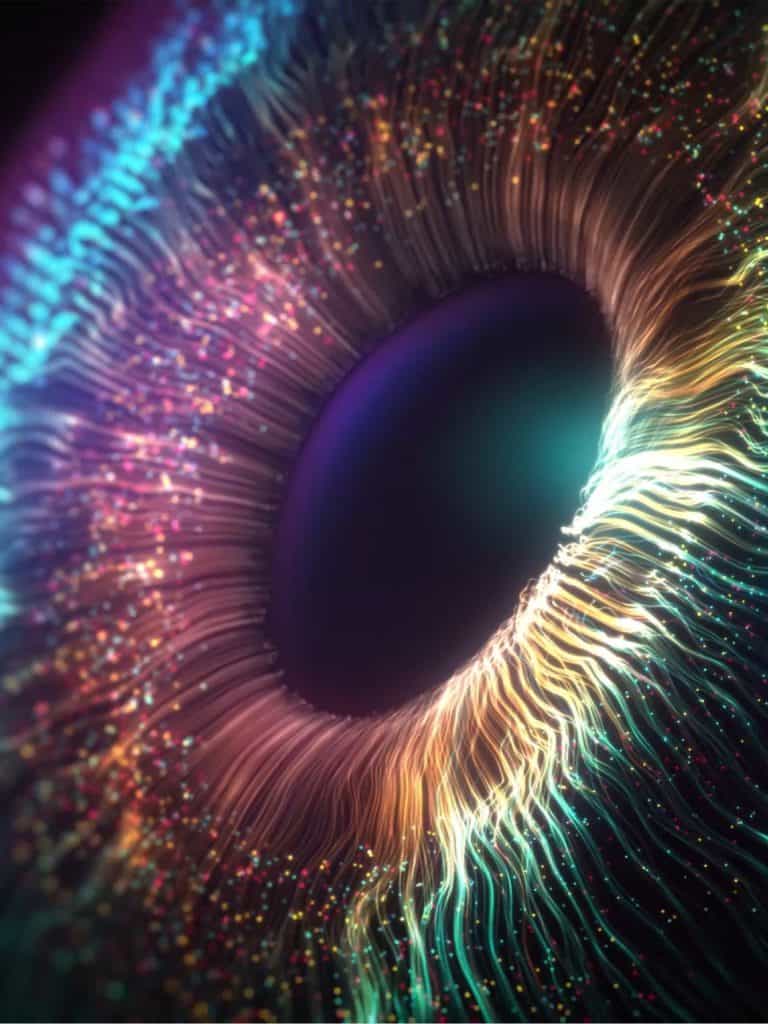
The training process in deep learning typically involves feeding large amounts of labeled data into the network, which learns progressively complex representations at each layer. This hierarchical learning enables deep learning models to excel at tasks like image recognition, natural language processing, and voice-based interactions. For example, deep learning powers facial recognition systems and voice assistants that understand natural language queries.
Similarities and Differences
Both machine learning and deep learning enable computers to learn from data, but they do so through different approaches and requirements. Both rely on training data to learn patterns and make predictions. However, deep learning often requires significantly larger datasets due to the complexity of neural networks and the resulting high number of model parameters to be optimized. Machine learning can use different algorithms, including deep learning, so they can be trained on both small and large datasets. Another distinction lies in interpretability—machine learning models not using deep learning are usually more interpretable, so we can more easily understand how they arrive at predictions, whereas the complexity of deep learning models can make it difficult to understand how and why certain predictions are made.
Machine Learning and Deep Learning: The Benefits and Limitations
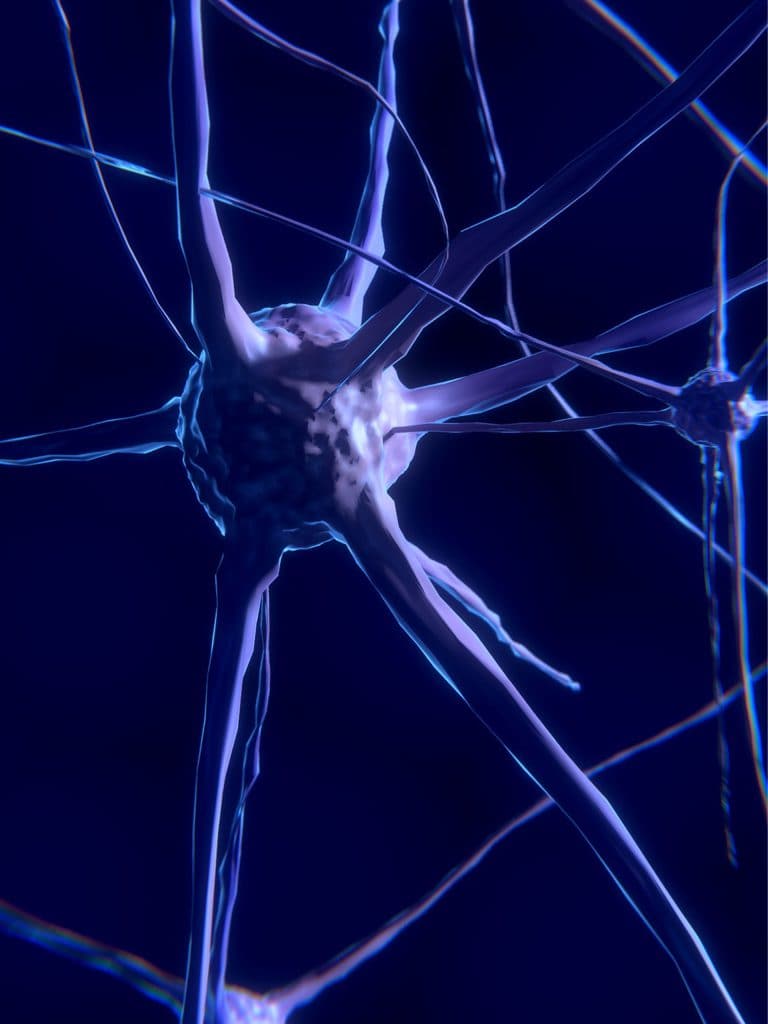
Because machine learning can be facilitated through deep learning, the line between the two can be blurred. For the purposes of this blog, we will refer to the benefits and limitations of machine learning when it does not use a deep learning algorithm.
Machine learning offers numerous benefits, including the ability to automate processes, identify patterns in complex data, and handle dynamic environments effectively. By continuously learning and adapting, machine learning algorithms can provide valuable insights and predictions for various applications. However, it’s important to note that machine learning models require periodic updates and retraining to stay accurate in a changing world and avoid bias issues inherent in the training data.
On the other hand, deep learning’s ability to process unstructured data makes it ideal for tasks such as image and speech recognition. Deep learning models can handle vast amounts of data and extract intricate features. However, deep learning models are computationally intensive (and expensive!) and require substantial computational resources for training, deployment, and updating.
Embracing the Power of Data with DataSeer
Both machine learning and deep learning have revolutionized the way we harness the power of data. By leveraging these technologies, organizations can uncover valuable insights, automate processes, and make data-driven decisions.
At DataSeer, we specialize in automated visualization software that digitizes unstructured data trapped in 2D images, creating a digital twin database with API access. Our innovative technology integrates industrial engineering with machine learning and computer vision, facilitating rapid project turnaround, automating change management tracking, and reducing risks associated with integrating legacy and greenfield processes. Whether it’s asset integrity management, plant cybersecurity, capital projects engineering, process simulations, or plant operations and maintenance, DataSeer empowers businesses to extract valuable information from unstructured data.
Collaborating with DataSeer allows organizations to accelerate their journey towards innovation by leveraging the power of machine learning and deep learning. Together, we can unlock the potential of data and drive industries forward into a future empowered by insights and intelligence. Contact us today to embark on this exciting journey!