Diving into the Depths of Machine Learning: Deep Learning vs Shallow Learning
In the ever-evolving field of machine learning, it is crucial to understand the nuances between different techniques. Previously, we explored the relationship between machine learning and deep learning. However, that exploration reserved another factor for this expedition: shallow learning. In this blog, we will delve into the depths of machine learning to define and compare two categories of it: deep and shallow learning. By examining their differences and benefits, we aim to provide a comprehensive understanding of these techniques. Additionally, we will highlight how DataSeer leverages both deep and shallow models to deliver outstanding performance to our users.
Defining Machine Learning
Before diving into the specifics of deep learning and shallow learning, let’s start by defining machine learning itself. At its core, machine learning is a subset of artificial intelligence which enables systems to automatically learn and improve from experience and from training or historical data without being explicitly programmed. It allows computers to analyze vast amounts of data and uncover meaningful patterns.
Shallow Learning
As a subset of machine learning techniques, shallow learning does not involve complex hierarchical layers of computation. These models are typically simpler and require less computational resources compared to deep learning models. Shallow learning algorithms, such as linear regression and support vector machines, focus on extracting patterns from input data without extensive layer-by-layer analysis. The effectiveness of shallow learning lies in its ability to quickly analyze and make predictions on relatively small datasets.
Deep Learning
In contrast to shallow learning, deep learning models are characterized by their deep neural networks, which consist of multiple layers of interconnected nodes. These models are capable of automatically learning highly intricate patterns and representations. Through “deep learning,” these neural networks can discern complex features from raw data, often achieving higher accuracy on challenging tasks like image recognition, natural language processing, and speech synthesis. Deep learning models, such as convolutional neural networks (CNNs) and recurrent neural networks (RNNs), have revolutionized fields such as computer vision and natural language processing.
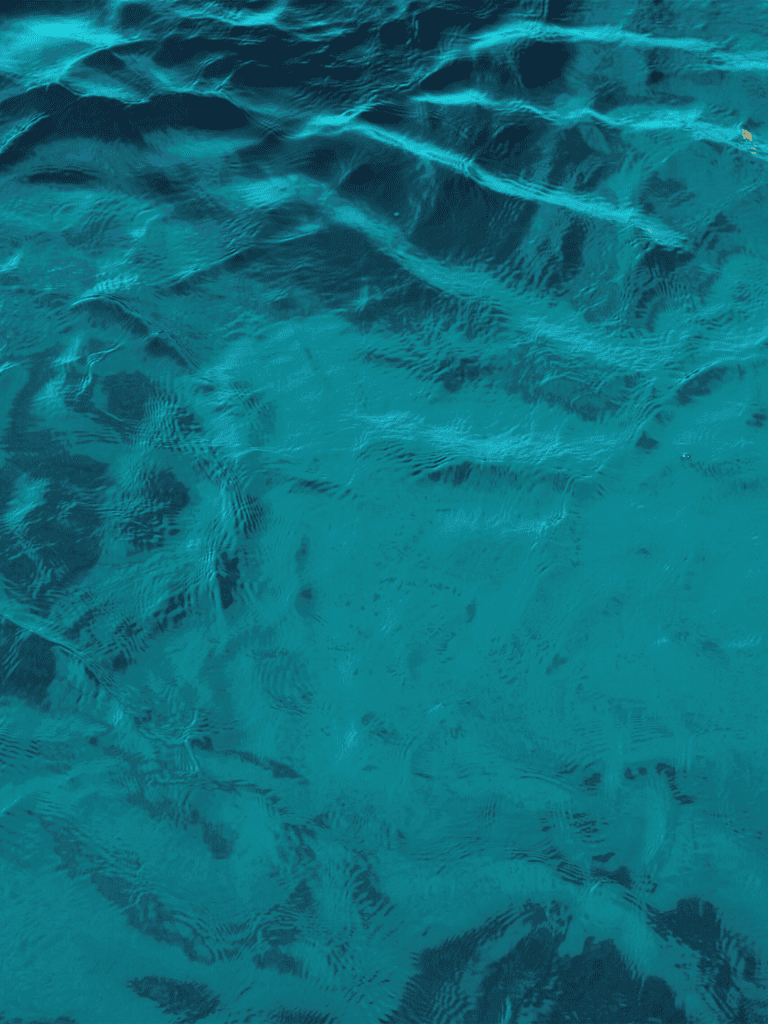
The Fundamental Technical Aspect: Deep Models vs Shallow Models
At the core of the distinction between deep learning and shallow learning lies the fundamental technical aspect: deep models vs. shallow models. Deep learning models directly address this aspect by introducing multiple layers of representation, enabling the extraction of higher-level features from raw data. This architectural difference influences various characteristics of the models, including the amount of training data required, interpretability, and computational resources. While deep learning models often require large amounts of training data and can be less interpretable, they excel at capturing complex dependencies and achieving high accuracy on a wide range of tasks.
DataSeer's Approach to Shallow and Deep Learning
DataSeer understands the significance of both deep and shallow learning in different scenarios. In our platform, we leverage deep learning models for our global symbol library. These models excel at extracting rich representations and recognizing complex patterns, allowing us to provide accurate results when digitizing unstructured data trapped in images.
We also utilize shallow models for real-time learning in our tool. These models are optimized for efficiency and speed, allowing for rapid analysis and quick insights. By combining the strengths of both deep and shallow learning models, DataSeer ensures users receive optimal performance for their specific needs.
Making Data Work for You
Exploring the depths of machine learning reveals the intricate relationship between deep learning and shallow learning. While shallow learning models offer speed and efficiency, deep learning models unlock the power to tackle complex tasks and achieve remarkable accuracy.
Machine learning continues to evolve, and exploring the possibilities of deep learning, shallow learning, and their integration provides exciting opportunities for data-driven professionals and businesses. As the field progresses, DataSeer remains committed to delivering cutting-edge solutions to harness the power of data to transform industries.
Remember, data holds the key to innovation, so let’s dive into the depths and unlock its potential together.