Material Takeoffs, Estimation & AI -- Top 4 Takeaways
On May 16, 2023, we hosted a webinar with estimation experts — Jaris Tilstra, Lead Estimator at Interstates, and Waymon Lofton, Director of Estimating at Black & Veatch — along with Jo-Anne Ting, CEO & President of DataSeer, to explore the state estimation & takeoffs in Capital Projects and how AI plays a role.
Conversation Top 4 Takeaways
#1
Capital projects today face challenges such as labor shortages due to a retiring workforce, high material costs, price fluctuations, supply chain disruptions and legislative regulations. Adoption of technology has been slow due to the conservative nature of the Engineering & Construction industry, but the recent rapid growth of E&C tech in the last 3 years show some appetite for disruption.
#2
When it comes to takeoffs, Jaris Tilstra from Interstates shared some challenges that contractors face. These include multiple symbolic representations (e.g. motors can be represented by the equipment they are driving); related pieces of data that don’t necessarily appear in a predictable pattern (for the example of motors, this can be horsepower & power type); and context— which is not always available, since design engineers who created the diagrams may not always follow standard conventions.
AI tools can help automate tedious tasks. An example he gave with DataSeer involved producing a takeoff of 3,500 rows from 100 pages in 20 min instead of days via a manual process. He emphasized that the human user is a key part of the automation process, though, to give context, interpret, and review final outputs.
How to get buy-in with new technologies? Fear of the unknown & job loss, lack of trust in software, data security or privacy concerns, a lack of knowledge and understanding of the underlying tech are all possible reasons for pushback. Understanding benefits, being confident in the ability to use it and feeling supported in the process are all key. So long as an AI tool can automate tedious takeoff tasks consistently and accurately, this allows the team to focus on more complex & creative work.
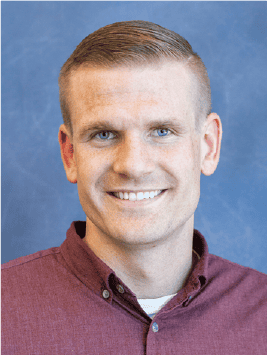
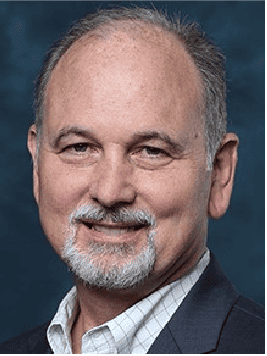
#3
Waymon Lofton from Black & Veatch described how customers today are pushing for a new realm of information. They want more accurate data. Per AACE standards, producing more accurate estimates is typically more expensive. Tools that produce accurate estimates that don’t cost more.. or, ideally, cost less is desirable.What’s so hard about getting accurate takeoffs? First, it’s not just about accuracy, it’s also about speed & getting things delivered in a timely fashion (ASAP!). If counts are wrong, pricing and construction are all wrong. It’s a cascading effect. Secondly, final doesn’t mean final. Engineers change things a lot, even after diagrams are “finalized”. Last minute changes need to be easily handled & incorporated into the estimation process.
There’s no shortcut to getting accurate estimates. No guessing. Necks are on the line. Waymon gave an example about how an AI tool like DataSeer could reduce time taken by a manual process of a team of people over a few weeks to 1-2 people over a few days (a time reduction of 90-95%). Beyond speed, there’s also less resources / fewer people needed. With a team, people could interpret things differently, causing errors in inconsistencies. With an AI tool, when errors were made, at least they were consistently made. Of course, it’s not like you can remove a user from the process since estimators need to review final data outputs.
What’s the bigger picture? There are plenty of AI tools out there to help with creating earlier, accurate estimates. Clients are demanding more, and the future for new AI tools is bright.
#4
Jo-Anne Ting from DataSeer unpacked some definitions for us (what’s AI, ML and Deep learning?) and covered key requirements for AI / ML to be applied successfully. These assumptions include i) access to labelled data so there’s a feedback loop on continued error correction; ii) the past being predictive of the future; and iii) making mistakes is ok and acceptable.She gave some examples of how AI tools have been developed across the Engineering & Construction lifecycle from pre-construction (e.g., software tools for data-driven estimation, supply chain optimization, project planning & controls for on-time and under budget delivery), construction (e.g., leveraging robotics & drone technology to ensure on-site safety and enhance worker productivity), and post-construction (e.g., tools for monitoring data integrity, asset performance management & predictive maintenance).
Things to watch out for? Some considerations with AI tools that everyone should be aware of: Who’s supplying & labeling the training data? What scenarios are acceptable for there to be mistakes? What do you do about data lying around that’s NOT structured and NOT labeled? How does collaboration between human + machine look like?
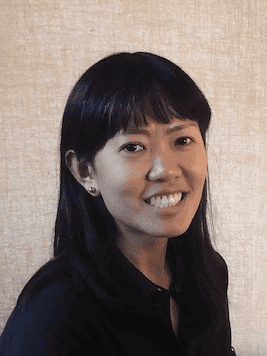